您现在的位置是:首页 >技术教程 >Pytorch从零开始实现Vision Transformer (from scratch)网站首页技术教程
Pytorch从零开始实现Vision Transformer (from scratch)
Pytorch从零开始实现Vision Transformer
前言
Transformer在NLP领域大放异彩,而实际上NLP(Natural Language Processing,自然语言处理)领域技术的发展都要先于CV(Computer Vision,计算机视觉),那么如何将Transformer这类模型也能适用到图像数据上呢?
在2017年Transformer发布后,历经3年时间,Vision Transformer于2020年问世。与Transformer相同,Vision Transformer也是由Google Brain和Google Research团队开发,然而并不是同一批人(除了Jakob Uszkoreit)。
值得一提的是,Vision Transformer并不是第一个将Transformer应用到CV上的,因为这些巨头的存在(如Google,FaceBook),论文的名气也自然会更大,而且从如今ViT的泛用程度来看也是,大家对其认可度更高纷纷follow。和这些巨头庞大资源比,高校产出的论文光芒显得黯淡了许多。而在大模型时代更是如此,都是“大力出奇迹”的结果。可大模型大数据训练就是AI的最终形态了吗,我觉得不然……或许在AI真正具有“智能”时,深度学习的模型也并不需要这么大吧,因为人脑正是有了联想推理才能拥有知识和技能,而不完全单靠记忆。
一、Vision Transformer架构介绍
1. Patch Embedding
2. Multi-Head Attention
3. Transformer Block
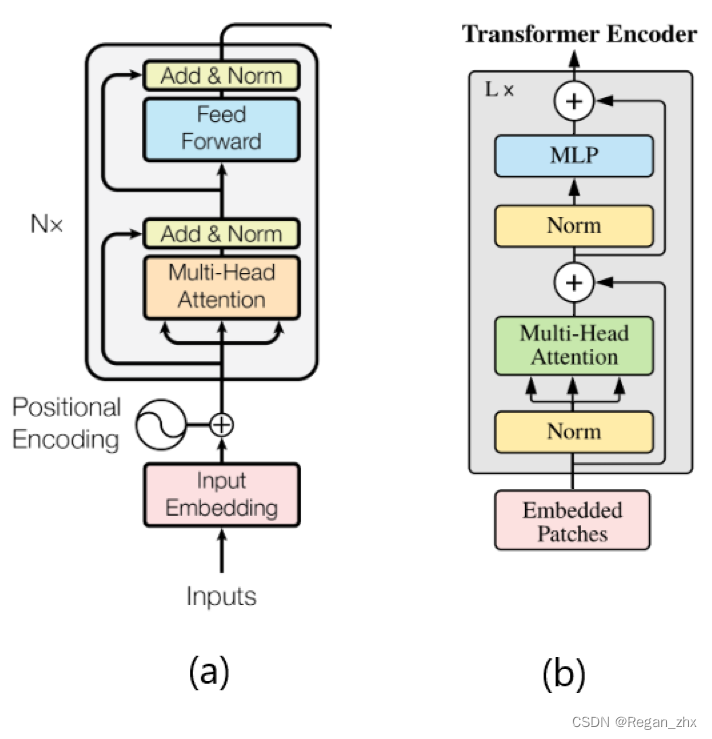
如图,(a) 是最初Transformer的Encoder结构图, (b)则是ViT的。可以明显看出,Transformer是在multi-head attention和feedforward模块后进行残差操作(即Add)和Norm(标准化),而ViT则是在这些模块前使用Norm操作。
Feed Forward
ViT的Feed Forward模块使用两层全连接层(Linear)和GeLU激活函数。而Transformer使用的是ReLu激活函数。
GeLu于2016年被提出,见于Bridging Nonlinearities and Stochastic Regularizers with Gaussian Error Linear Units,后来经过论文修改改名为“Gaussian Error Linear Units (GELUs)”。论文给出了ReLu和GeLu的图示:
ReLu确实好用,但缺点也很明显,其在输入值小于0时都会输出0,这样“一刀切”的策略势必会丢掉信息,累计error。因此后来出现了GeLu、LeakyReLu等一系列激活函数来解决神经元”死亡“问题,让输入值小于0时输出不总是0。
二、预备知识
本节的两个操作都是为了方便编程人员更好对tensor进行操作,且让代码更具可读性。
1. Einsum
Einsum即爱因斯坦和,torch.einsum即可调用。
2. Einops
是大牛 受Einsum启发所开发的一个库,主要用于张量的变形等操作。
三、Vision Transformer代码实现
这次代码并不是直接取用某一份代码,而是参考包括Pytorch官方的代码库、网上博客、github项目综合出的一份Vision Transformer代码,尽可能还原ViT又兼顾代码可读性以便读者学习理解。此处引用比ViT原论文更加具体的ViT模型图:
此图出自论文Vision Transformers for Remote Sensing Image Classification。
0. 导入库
import torch
import torch.nn as nn
from einops import rearrange, repeat
from einops.layers.torch import Rearrange
1. Patch Embedding
class PatchEmbedding(nn.Module):
def __init__(self, embed_size=768, patch_size=16, channels=3, img_size=224):
super(PatchEmbedding, self).__init__()
self.patch_size = patch_size
# Version 1.0
# self.patch_projection = nn.Sequential(
# Rearrange("b c (h h1) (w w1) -> b (h w) (h1 w1 c)", h1=patch_size, w1=patch_size),
# nn.Linear(patch_size * patch_size * channels, embed_size)
# )
# Version 2.0
self.patch_projection = nn.Sequential(
nn.Conv2d(channels, embed_size, kernel_size=(patch_size, patch_size), stride=(patch_size, patch_size)),
Rearrange("b e (h) (w) -> b (h w) e"),
)
self.cls_token = nn.Parameter(torch.randn(1, 1, embed_size))
self.positions = nn.Parameter(torch.randn((img_size // patch_size) ** 2 + 1, embed_size))
def forward(self, x):
batch_size = x.shape[0]
x = self.patch_projection(x)
# prepend the cls token to the input
cls_tokens = repeat(self.cls_token, "() n e -> b n e", b=batch_size)
x = torch.cat([cls_tokens, x], dim=1)
# add position embedding
x += self.positions
return x
2. Residual & Norm
class Residual(nn.Module):
def __init__(self, fn):
super(Residual, self).__init__()
self.fn = fn
def forward(self, x, **kwargs):
return self.fn(x, **kwargs) + x
class PreNorm(nn.Module):
def __init__(self, dim, fn):
super(PreNorm, self).__init__()
self.norm = nn.LayerNorm(dim)
self.fn = fn
def forward(self, x, **kwargs):
return self.fn(self.norm(x), **kwargs)
3. Multi-Head Attention & FeedForward
class FeedForward(nn.Module):
def __init__(self, dim, hidden_dim, dropout=0.):
super(FeedForward, self).__init__()
self.mlp = nn.Sequential(
nn.Linear(dim, hidden_dim),
nn.GELU(),
nn.Dropout(dropout),
nn.Linear(hidden_dim, dim),
nn.Dropout(dropout),
)
def forward(self, x):
return self.mlp(x)
class MultiHeadAttention(nn.Module):
def __init__(self, embed_dim=768, n_heads=8, dropout=0.):
"""
Args:
embed_dim: dimension of embeding vector output
n_heads: number of self attention heads
"""
super(MultiHeadAttention, self).__init__()
self.embed_dim = embed_dim # 768 dim
self.n_heads = n_heads # 8
self.head_dim = self.embed_dim // self.n_heads # 768/8 = 96. each key,query,value will be of 96d
self.scale = self.head_dim ** -0.5
self.attn_drop = nn.Dropout(dropout)
# key,query and value matrixes
self.to_qkv = nn.Linear(self.embed_dim, self.embed_dim * 3, bias=False)
self.to_out = nn.Sequential(
nn.Linear(self.embed_dim, self.embed_dim),
nn.Dropout(dropout)
)
def forward(self, x):
"""
Args:
x : a unified vector of key query value
Returns:
output vector from multihead attention
"""
qkv = self.to_qkv(x).chunk(3, dim=-1)
q, k, v = map(lambda t: rearrange(t, "b n (h d) -> b h n d", h=self.n_heads), qkv)
dots = torch.einsum('bhid,bhjd->bhij', q, k) * self.scale
attn = dots.softmax(dim=-1)
attn = self.attn_drop(attn)
out = torch.einsum('bhij,bhjd->bhid', attn, v)
out = rearrange(out, "b h n d -> b n (h d)")
out = self.to_out(out)
return out
4. Transformer Encoder
class Transformer(nn.Module):
def __init__(self, dim=768, depth=12, n_heads=8, mlp_expansions=4, dropout=0.):
super(Transformer, self).__init__()
self.layers = nn.ModuleList([])
for _ in range(depth):
self.layers.append(nn.ModuleList([
Residual(PreNorm(dim, MultiHeadAttention(dim, n_heads, dropout))),
Residual(FeedForward(dim, dim * mlp_expansions, dropout))
]))
def forward(self, x):
for attn, ff in self.layers:
x = attn(x)
x = ff(x)
return x
6. Vision Transformer
class VisionTransformer(nn.Module):
def __init__(self, dim=768,
patch_size=16,
channels=3,
img_size=224,
depth=12,
n_heads=8,
mlp_expansions=4,
dropout=0.,
num_classes=0,
global_pool='avg'):
super(VisionTransformer, self).__init__()
assert global_pool in ('avg', 'token')
self.global_pool = global_pool
self.patch_embedding = PatchEmbedding(dim, patch_size, channels, img_size)
self.transformer = Transformer(dim, depth, n_heads, mlp_expansions, dropout)
self.mlp_head = nn.Sequential(
nn.LayerNorm(dim),
nn.Linear(dim, num_classes)
) if num_classes > 0 else nn.Identity()
def forward(self, img):
x = self.patch_embedding(img)
x = self.transformer(x)
x = x[:, 1:].mean(dim=1) if self.global_pool == 'avg' else x[:, 0]
x = self.mlp_head(x)
return x
7. Test Code
if __name__ == '__main__':
device = torch.device("cuda" if torch.cuda.is_available() else "cpu")
images = torch.randn((16, 3, 224, 224)).to(device)
vit = VisionTransformer(num_classes=4, global_pool="token").to(device)
output = vit(images)
print(output)
torch.save(vit.state_dict(), "model.pth")
模型参数量计算
1. 卷积核参数量计算
对于二维卷积层,其参数量由输入通道数(C)、卷积核的大小(KxK)、卷积核的数量或者说输出通道数(F)、偏置项的数量等因素决定。计算公式为:
(
K
×
K
×
C
+
1
)
×
F
(K imes K imes C + 1) imes F
(K×K×C+1)×F,其中1为偏置项。
2. 全连接层参数量计算
对于某一层全连接层的参数量只由其输入维度和输出维度(是否带偏置项)决定,将全连接层理解为一个映射函数,假设输入为矩阵A(维度为HxW),输出为矩阵C(维度为HxH),那么一层全连接层参数量就来自其所代表的矩阵B根据矩阵乘法其维度应为WxH,即Linear(W,H),输入维度W,输出维度也是H。计算公式易得:
W
×
H
+
H
×
1
W imes H + H imes 1
W×H+H×1,其中1代表偏置项,需要输出维度个偏置项。
3. ViT参数量计算
模块/变量名 | 计算过程 | 参数量 |
---|---|---|
PatchEmbedding | c o n v 2 d + c l s _ t o k e n + p o s t i t i o n s conv2d + cls\_token + postitions conv2d+cls_token+postitions | 742656 |
conv2d | ( 16 × 16 × 3 + 1 ) × 768 (16 imes 16 imes 3 + 1) imes 768 (16×16×3+1)×768 | 590592 |
cls_token | 1 × 1 × 768 1 imes1 imes768 1×1×768 | 768 |
postitions | ( ( 224 ÷ 16 ) 2 + 1 ) × 768 ((224div 16)^2+1) imes768 ((224÷16)2+1)×768 | 151296 |
Feedforward | ( 768 × ( 768 × 4 ) + ( 768 × 4 ) ) + ( ( 768 × 4 ) × 768 + 768 ) (768 imes(768 imes4)+(768 imes4)) + ((768 imes4) imes768+768) (768×(768×4)+(768×4))+((768×4)×768+768) | 4722432 |
MultiHeadAttention | t o _ q k v + t o _ o u t to\_qkv + to\_out to_qkv+to_out | 2360064 |
to_qkv | 768 × ( 768 × 3 ) 768 imes(768 imes3) 768×(768×3) | 1769472 |
to_out | 768 × 768 + 768 768 imes768+768 768×768+768 | 590592 |
Transformer | 12 × ( F e e d f o r w a r d + M u l t i H e a d A t t e n t i o n ) 12 imes(Feedforward+MultiHeadAttention) 12×(Feedforward+MultiHeadAttention) | 84989952 |
ViT | T r a n s f o r m e r + P a t c h E m b e d d i n g + m l p _ h e a d Transformer+PatchEmbedding+mlp\_head Transformer+PatchEmbedding+mlp_head | 85735684 |
mlp_head | 768 × n u m _ c l a s s e s + n u m _ c l a s s e s ,本文设置 n u m _ c l a s s e s 为 4 768 imes num\_classes+num\_classes,本文设置num\_classes为4 768×num_classes+num_classes,本文设置num_classes为4 | 3076 |
最终参数量为
85735684
×
4
(
B
)
=
342942736
(
B
)
85735684 imes 4(B) = 342942736(B)
85735684×4(B)=342942736(B),为什么要乘以4字节呢?
因为这些参数权重默认为float32保存,需要用到32bits即4Bytes,最终通过换算得,
342942736
(
B
)
÷
1024
÷
1024
=
327.055679321
(
M
B
)
342942736(B)div 1024div 1024 = 327.055679321(MB)
342942736(B)÷1024÷1024=327.055679321(MB)
因为我们在Test code有保存模型权重为model.pth文件,可以查看model.pth属性来验证计算是否准确。
在字节数上有所偏差,但足以表明计算过程大致是正确的! 偏差可能原因是model.pth不止要保存权重,还会附带一些其他信息,所以实际文件大小会比参数量要略大。
总结
日志
参考文献
https://theaisummer.com/vision-transformer/
https://medium.com/mlearning-ai/vision-transformers-from-scratch-pytorch-a-step-by-step-guide-96c3313c2e0c
https://www.kaggle.com/code/hannes82/vision-transformer-trained-from-scratch-pytorch
https://towardsdatascience.com/implementing-visualttransformer-in-pytorch-184f9f16f632
https://github.com/FrancescoSaverioZuppichini/ViT